Analisis Teks Pemberitaan Telemedicine di Indonesia: Pendekatan Sentimen, NER, Topic Modeling, dan Social Network dalam Memahami Isu dan Persepsi
Abstract
Abstract
Telemedicine is becoming an increasingly relevant phenomenon in the health sector in Indonesia, especially with the emergence of the COVID-19 Pandemic. This study examines text analysis of telemedicine news coverage during the COVID-19 pandemic in Indonesia using sentiment analysis, Named Entity Recognition (NER), topic modeling, and Social Network Analysis (SNA). This research aims to gain an in-depth understanding of issues, public perceptions, social networks, and topics related to the use of telemedicine in dealing with a pandemic. This study provides a comprehensive understanding of telemedicine coverage during the COVID-19 pandemic in Indonesia by combining four methods. The findings of this research can provide valuable insights for stakeholders in optimizing the use of telemedicine, understanding public perceptions, and building effective collaborations in handling pandemics.
Keywords: telemedicine, sentiment analysis, Named Entity Recognition (NER), topic modeling, social network analysis, COVID-19
Abstrak
Telemedicine menjadi fenomena yang semakin relevan dalam sektor kesehatan di Indonesia, terutama dengan munculnya Pandemi COVID-19. Penelitian ini mengkaji analisis teks pemberitaan telemedicine selama pandemi COVID-19 di Indonesia dengan menggunakan analisis sentimen, Named Entity Recognition (NER), Topic Modeling, dan Social Network Analysis (SNA). Tujuan penelitian ini adalah untuk memperoleh pemahaman yang mendalam tentang isu-isu, persepsi masyarakat, jaringan sosial, dan topik-topik yang terkait dengan pemanfaatan telemedicine dalam menghadapi masalah kesehatan di masa pandemi. Penggunaan gabungan empat metode analisis agar dapat menyajikan pemahaman yang komprehensif tentang pemberitaan telemedicine selama pandemi COVID-19 di Indonesia. Hasil penelitian menunjukkan adanya kecenderungan sentimen positif dan netral terhadap telemedicine dan keberadaannya sangat membantu masalah kesehatan di masa Pandemi COVID-19. Selain itu pejabat pemerintah adalah nama yang paling sering muncul dalam pemberitaan telemedicine yang memiliki makna peranan sentral pemerintah dalam masalah kesehatan sangat dibutuhkan. Penelitian ini diharapkan dapat memberikan wawasan berharga bagi para pemangku kepentingan dalam mengoptimalkan pemanfaatan telemedicine, memahami persepsi masyarakat, dan membangun kolaborasi yang efektif dalam penanganan pandemi.
Kata Kunci: telemedicine, analisis sentimen, Named Entity Recognition (NER), social network analysis, topic modelling, COVID-19
Keywords
Full Text:
PDFReferences
A. E. Loeb, S. S. Rao, J. R. Ficke, C. D. Morris, L. H. Riley, and A. S. Levin, “Departmental Experience and Lessons Learned With Accelerated Introduction of Telemedicine During the COVID-19 Crisis,” Journal of the American Academy of Orthopaedic Surgeons, vol. 28, no. 11, pp. e469–e476, Jun. 2020, doi: 10.5435/JAAOS-D-20-00380.
R. Bashshur, C. R. Doarn, J. M. Frenk, J. C. Kvedar, and J. O. Woolliscroft, “Telemedicine and the COVID-19 Pandemic, Lessons for the Future,” Telemedicine and e-Health, vol. 26, no. 5, pp. 571–573, May 2020, doi: 10.1089/tmj.2020.29040.rb.
F. F. Rachman and S. Pramana, “Analisis Sentimen Pro dan Kontra Masyarakat Indonesia tentang Vaksin COVID-19 pada Media Sosial Twitter,” Jakarta: Lembaga Penerbitan Universitas Esa Unggul, 2020.
V. Mahajan, T. Singh, and C. Azad, “Using Telemedicine During the COVID-19 Pandemic,” Indian Pediatr, vol. 57, no. 7, pp. 658–661, Jul. 2020, doi: 10.1007/s13312-020-1895-6.
Kementerian Kesehatan RI, Pedoman Pelayanan Kesehatan Melalui Telemedicine pada Masa Pandemi Corona Virus Disease 2019 (COVID 19). Jakarta: Kementerian Kesehatan RI, 2021.
K. Afifah, I. N. Yulita, and I. Sarathan, “Sentiment Analysis on Telemedicine App Reviews using XGBoost Classifier,” in 2021 International Conference on Artificial Intelligence and Big Data Analytics, IEEE, Oct. 2021, pp. 22–27. doi: 10.1109/ICAIBDA53487.2021.9689762.
E. Kang, N. Song, and H. Ju, “Contents and sentiment analysis of newspaper articles and comments on telemedicine in Korea: Before and after of COVID-19 outbreak,” Health Informatics J, vol. 28, no. 1, p. 146045822210755, Jan. 2022, doi: 10.1177/14604582221075549.
H. Herwando and T. H. Sitompul, “Evaluasi Manfaat Penerapan Telemedicine di Negara Kepulauan: Systematic Literature Review,” Indonesian of Health Information Management Journal (INOHIM), vol. 9, no. 2, pp. 91–101, Dec. 2021, doi: 10.47007/inohim.v9i2.261.
N. Dzulvawan and S. Pramana, “Pemetaan Kesiapan Penerapan Telemedika di Indonesia,” Indonesian of Health Information Management Journal (INOHIM), vol. 10, no. 2, pp. 118–125, Dec. 2022, doi: 10.47007/inohim.v10i2.436.
D. Krismawati, W. C. F. Mariel, F. A. Arsyi, and S. Pramana, “Sentiment Analysis on Indonesia Economic Growth using Deep Learning Neural Network Method,” Korea Distribution Science Association, 2022.
H. Talpada, M. N. Halgamuge, and N. Tran Quoc Vinh, “An Analysis on Use of Deep Learning and Lexical-Semantic Based Sentiment Analysis Method on Twitter Data to Understand the Demographic Trend of Telemedicine,” in 2019 11th International Conference on Knowledge and Systems Engineering (KSE), IEEE, Oct. 2019, pp. 1–9. doi: 10.1109/KSE.2019.8919363.
D. N. Myers and J. W. McGuffee, “Choosing Scrapy,” 2015.
B. Pang and L. Lee, “Opinion Mining and Sentiment Analysis,” Foundations and Trends® in Information Retrieval, vol. 2, no. 1–2, pp. 1–135, 2008, doi: 10.1561/1500000011.
D. Nadeau and S. Sekine, “A survey of named entity recognition and classification,” Lingvisticae Investigationes, vol. 30, no. 1, pp. 3–26, Aug. 2007, doi: 10.1075/li.30.1.03nad.
W. Wahyudin, “Aplikasi Topic Modeling Pada Pemberitaan Portal Berita Online Selama Masa Psbb Pertama,” Seminar Nasional Official Statistics, vol. 2020, no. 1, pp. 309–318, Jan. 2021, doi: 10.34123/semnasoffstat.v2020i1.579.
D. M. Blei, “Probabilistic topic models,” Commun ACM, vol. 55, no. 4, pp. 77–84, Apr. 2012, doi: 10.1145/2133806.2133826.
J. Scott, “Social Network Analysis,” Sociology, vol. 22, no. 1, pp. 109–127, Feb. 1988, doi: 10.1177/0038038588022001007.
Chaves A, “Scrapy Documentation Release 2.5.0 Scrapy developers,” 2021. Accessed: Jul. 19, 2021. [Online]. Available: https://docs.scrapy.org/en/latest
S. Satriajati, S. B. Panuntun, and S. Pramana, “Implementasi Web Scraping dalam Pengumpulan Berita Kriminal pada Masa Pandemi Covid-19,” Seminar Nasional Official Statistics, vol. 2020, no. 1, pp. 300–308, Jan. 2021, doi: 10.34123/semnasoffstat.v2020i1.578.
D. H. Wahid and A. SN, “Peringkasan Sentimen Esktraktif di Twitter Menggunakan Hybrid TF-IDF dan Cosine Similarity,” IJCCS (Indonesian Journal of Computing and Cybernetics Systems), vol. 10, no. 2, p. 207, Jul. 2016, doi: 10.22146/ijccs.16625.
DOI: https://doi.org/10.47007/inohim.v11i1.500
Refbacks
- There are currently no refbacks.
Lembaga Penerbitan Universitas Esa Unggul
Jl Arjuna Utara No 9. Tol Tomang, Kebon Jeruk, Jakarta. 11510
Email : inohim.ueu@esaunggul.ac.id
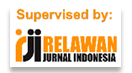
This work is licensed under a Creative Commons Attribution-NonCommercial 4.0 International License.
View My Stats